class: split-70 bg-main1 hide-slide-number right count: false .column[.vmiddle[ .font3[A Gentle Introduction to .amber[Biostatistics]] ]] .column.bg-main4[.vmiddle[.left[ .font1[ .amber[Aly Lamuri] Indonesia Medical Education and Research Institute ] ]]] --- layout: true class: split-two bg-main3 .column.bg-white[.vmiddle.center[ {{content}} ]] .column[.vmiddle.split-nine[.content[ # About me .row[.content[ Aly Lamuri ]] .row[.content[ FKUI 2011 ]] .row[.content[ Newcastle 2018 ]] .row[.content[ Academic writer ]] .row[.content[ Research assistant ]] ]]] --- class: fade-row2-col2 fade-row3-col2 fade-row4-col2 fade-row5-col2 <img src="https://dinkespapuabarat.files.wordpress.com/2018/01/e7b65479b4b0d73d0d93d77eebecace72c9ed142-e1516576360811.jpeg?w=736" width="100%"> --- class: fade-row3-col2 fade-row4-col2 fade-row5-col2 count: false <img src="https://axeldefifthseries.files.wordpress.com/2011/12/2011-maju-edited.jpg" width="100%"> --- class: fade-row4-col2 fade-row5-col2 count: false <img src="https://www.studyabroad.sg/site/wp-content/uploads/img_5a2c9ca986ab9.jpg" width="100%"> --- class: fade-row5-col2 count: false <img src="https://scontent-sin6-1.xx.fbcdn.net/v/t1.0-9/p960x960/34506502_1715646061858593_2631786956320669696_o.jpg?_nc_cat=100&_nc_sid=85a577&_nc_eui2=AeEp7DfkXrB3GDV0em7QdQvqBOuoUeR3T6ME66hR5HdPozAFZvxw6glNGL0SVQEY8s8&_nc_ohc=AGdfUOW3Xn4AX89FUUD&_nc_ht=scontent-sin6-1.xx&tp=6&oh=ee070b1cd9ab8e99db92461ba456518d&oe=5F790351" width="100%"> --- count: false <img src="https://upload.wikimedia.org/wikipedia/id/6/64/Makara-ui-fk.png" height="100%"> --- layout: false class: split-30 bg-main2 hide-slide-number count: false .column[.vmiddle[.right[ .font4[.amber[Outline]] ]]] .column.bg-main4[.vmiddle[.font2[ - .amber[Lecture overview] - Parameters in population - Statistics in acquired samples - Descriptive statistics ]]] --- layout: true class: bg-main3 split-two .column[.vmiddle.center[ {{content}} ]] .column[.vmiddle.split-three[.content[ .row[.content[ # Overview - Three hours of lecture? .red[Please, no.] - Session: lecture, Q&A, journal discussion - .amber[Fourteen] sessions in total ]] .row[.content[ ## Aim Understand the .amber[basic] of statistics ]] .row[.content[ ## .amber[Objectives] - Types of data and distribution - Test of differences - Correlation - Simple linear model ]] ]]] --- class: hide-row2-col2 hide-row3-col2 ??? - Encourage active participation (+icebreaking) - Use zoom feature: raise hand, voice chat, etc - Kahoot! --- class: hide-row3-col2 count: false <img src="https://i.pinimg.com/originals/e2/f7/ce/e2f7ce779432e38e9f75f72d328dd581.png" width="100%"> --- count: false <img src="https://i.pinimg.com/originals/e2/f7/ce/e2f7ce779432e38e9f75f72d328dd581.png" width="100%"> --- layout: false class: bg-main3 font2 # Agreement - Attend .amber[all] lectures (at minimum: .red[80%]) -- - Be .amber[on time], even if you are late make it no longer than .red[10 minutes] -- - Turn on the .amber[front camera] during the beginning of each lecture. -- And smile, because I'm taking a screenshot of your attendance :) -- - Pay .amber[attention] during classes. -- I may randomly ask you a question -- - .amber[Actively] participate in class -- - You may ask for .amber[permission] to temporarily leave the class. -- But .red[please return], because the class is gonna miss you :( -- - Turn on the front camera during .amber[presentation] -- - Do the .amber[assignment] ??? Show the course layout https://docs.google.com/document/d/1IE1H79-IQviC-vbHFSHvmMJNDbM8eiqZS69DkGHkPrY/edit?usp=sharing --- class: bg-main3 middle center .amber.font5[HBU?] ??? - What's your expectation in attending this biostatistics course? --- class: split-30 bg-main2 hide-slide-number layout: false count: false .column[.vmiddle[.right[ .font4[.amber[Outline]] ]]] .column.bg-main4[.vmiddle[.font2[ - Lecture overview - .amber[Parameters in population] - .red[Statistics in acquired samples] - Descriptive statistics ]]] --- class: bg-main3 middle split-two ## Population .amber[All] observable subjects inhabiting a certain location <br> -- ## Parameters Quantitative .amber[summary] of a population <br> -- ## Be more .amber[specific,] please? -- .column[ ] .column[.vmiddle[ ### Notation and meanings `\(X\)`: Data element `\(N\)`: Number of element `\(P\)`: Proportion `\(M\)`: .yellow[Median] `\(\mu\)`: .yellow[Average] `\(\sigma\)`: .yellow[Standard deviation] `\(\sigma^2\)`: .yellow[Variance] `\(\rho\)`: Correlation coefficient ]] --- class: bg-main3 middle split-two ## Sample A .red[subset] of an observable population <br> -- ## Statistics Quantitative .red[summary] of a sample <br> -- ## Be more .red[specific,] please? -- .column[ ] .column[.vmiddle[ ### Notation and meanings `\(x\)`: Data element `\(n\)`: Number of element `\(p\)`: Proportion `\(m\)`: .deep-orange[Median] `\(\bar{x}\)`: .deep-orange[Average] `\(s\)`: .deep-orange[Standard deviation] `\(s^2\)`: .deep-orange[Variance] `\(r\)`: Correlation coefficient ]] --- class: split-30 center bg-main3 .row.bg-main2[.vmiddle[ # Spot the differences! ]] .row[ <br> <table class=" lightable-minimal" style='font-family: "Trebuchet MS", verdana, sans-serif; width: auto !important; margin-left: auto; margin-right: auto;'> <thead> <tr> <th style="text-align:left;"> Statistics </th> <th style="text-align:left;"> Meanings </th> <th style="text-align:left;"> Parameters </th> </tr> </thead> <tbody> <tr> <td style="text-align:left;"> `\(x\)` </td> <td style="text-align:left;"> Data element </td> <td style="text-align:left;"> `\(X\)` </td> </tr> <tr> <td style="text-align:left;"> `\(n\)` </td> <td style="text-align:left;"> Number of element </td> <td style="text-align:left;"> `\(N\)` </td> </tr> <tr> <td style="text-align:left;"> `\(p\)` </td> <td style="text-align:left;"> Proportion </td> <td style="text-align:left;"> `\(P\)` </td> </tr> <tr> <td style="text-align:left;"> `\(m\)` </td> <td style="text-align:left;"> Median </td> <td style="text-align:left;"> `\(M\)` </td> </tr> <tr> <td style="text-align:left;"> `\(\bar{x}\)` </td> <td style="text-align:left;"> Average </td> <td style="text-align:left;"> `\(\mu\)` </td> </tr> <tr> <td style="text-align:left;"> `\(s\)` </td> <td style="text-align:left;"> Standard deviation </td> <td style="text-align:left;"> `\(\sigma\)` </td> </tr> <tr> <td style="text-align:left;"> `\(s^2\)` </td> <td style="text-align:left;"> Variance </td> <td style="text-align:left;"> `\(\sigma^2\)` </td> </tr> <tr> <td style="text-align:left;"> `\(r\)` </td> <td style="text-align:left;"> Correlation coefficient </td> <td style="text-align:left;"> `\(\rho\)` </td> </tr> </tbody> </table> ] --- class: split-30 bg-main2 hide-slide-number layout: false count: false .column[.vmiddle[.right[ .font4[.amber[Outline]] ]]] .column.bg-main4[.vmiddle[.font2[ - Lecture overview - Parameters in population - Statistics in acquired samples - .amber[Descriptive statistics] ]]] --- class: bg-main3 # Data Element - Suppose we have height data from a particular student group, denoted by `\(X\)` -- - Let `\(X \ni \{x_1, x_2, ..., x_n\}\)` -- - Then each of `\(\{x_1, x_2, ..., x_n\}\)` is the corresponding .amber[element] of the data -- - .amber[Easy, right? ] Let's see some numbers. -- ```r set.seed(1) *X <- rnorm(10, mean=160, sd=10) print(X) ``` ``` ## [1] 153.7 161.8 151.6 176.0 163.3 151.8 164.9 167.4 165.8 156.9 ``` -- ```r print(X[7]) ``` ``` ## [1] 164.9 ``` --- class: bg-main3 # Number of Element - From previous example, we understood `\(x_{1...n} \in X\)` -- - ...and each `\(x\)` as an element data -- - But what about `\(n\)`? -- - Turns out, `\(n\)` is the total number of .amber[element]! -- - A quick demo: ```r length(X) ``` ``` ## [1] 10 ``` --- class: bg-main3 # Proportion - From our synthetic data, we observed some people with a taller stature -- - Let 165 be a threshold, where people above 165 cm considered as tall -- - Then we can compute the .amber[proportion] of tall people compared to (*ahem*) not-so-tall people -- - So, we can simply conclude: `\(p = \frac{g}{n},\ where:\)` `\(p\)`: Proportion `\(g\)`: Group of interest `\(n\)`: Number of element -- - So, how many tall people do we have in our data? ```r set.seed(1) *X <- rnorm(10, mean=160, sd=10) print(X) ``` ``` ## [1] 153.7 161.8 151.6 176.0 163.3 151.8 164.9 167.4 165.8 156.9 ``` -- ```r sum(X > 165) / length(X) ``` ``` ## [1] 0.3 ``` --- layout: true class: bg-main3 split-30 # Mean - Assume: data presents an even distribution - Meaning that, data has a roughly **equal number of observation** on both ends - We can calculate the .amber[average] value as a general description, with: `$$\bar{x} = \frac{1}{n}\displaystyle \sum_{i=1}^{n} x_i$$` -- .row[.content[ ]] .row[.content[ {{content}} ]] --- --- count: false - In action: ```r *sum(X) / length(X) ``` ``` ## [1] 161.3 ``` --- count: false - In action: ```r sum(X) / length(X) ``` ``` ## [1] 161.3 ``` ```r *mean(X) ``` ``` ## [1] 161.3 ``` --- count: false - In action: ```r sum(X) / length(X) ``` ``` ## [1] 161.3 ``` ```r mean(X) ``` ``` ## [1] 161.3 ``` .font2[**Problem:** Not all data *distributed evenly*] --- count: false On a quick glimpse: 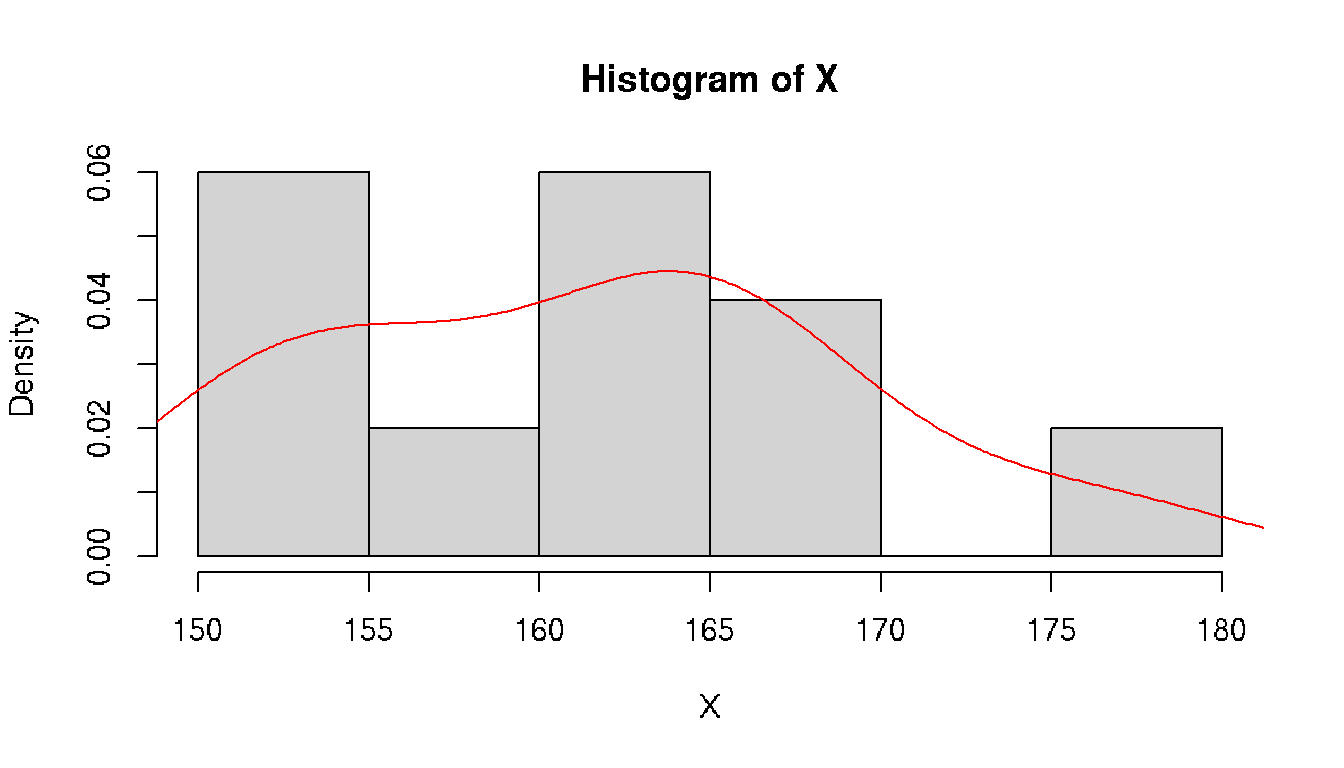<!-- --> --- count: false **Solution:** Use another measure `\(\to\)` median 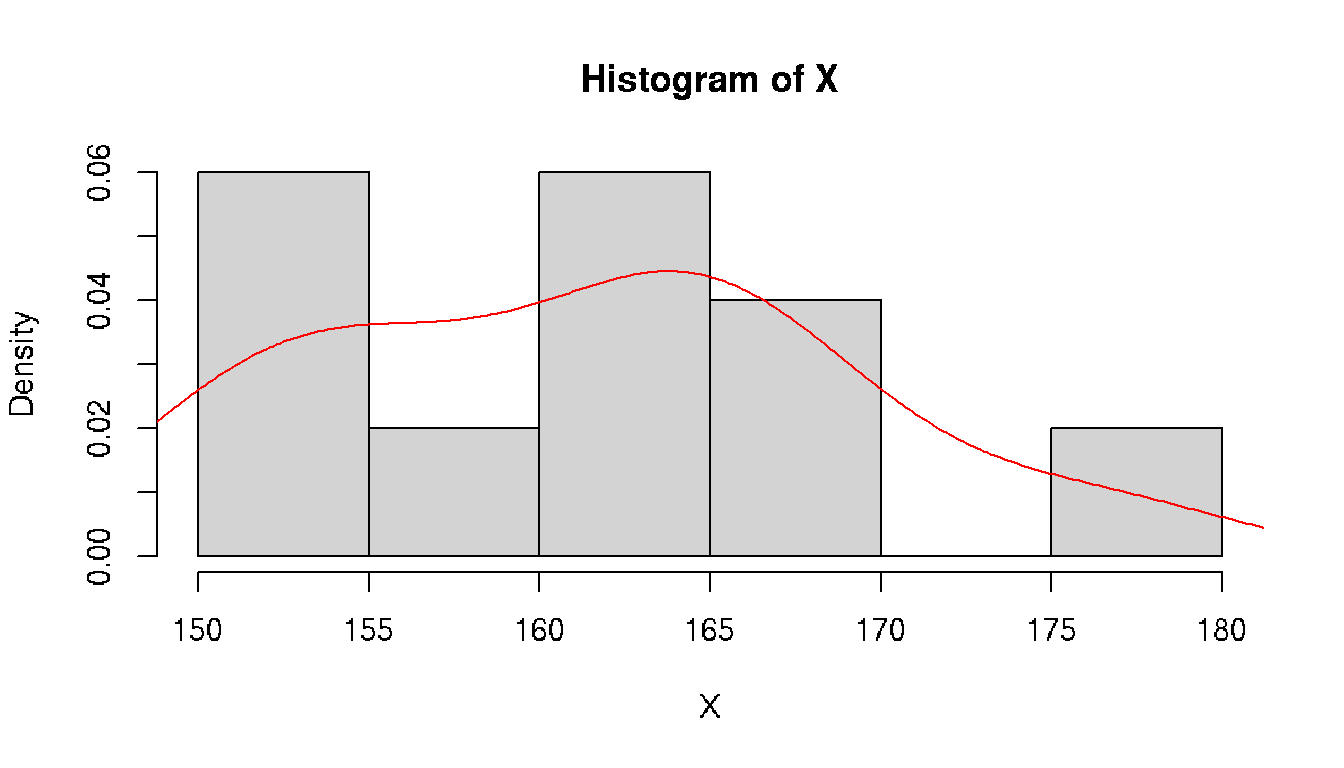<!-- --> --- layout: false class: bg-main3 # Median - Sort data ascendingly - Find the mid point of such data - Median is the **middle** index -- - Or, .amber[mathematically]: `$$m = \begin{cases} x_{\frac{n+1}{2}} & :n_{2\nmid} \\ \frac{1}{2} \left(x_{\frac{n}{2}} + x_{\frac{n}{2} + 1} \right) & :n_{2\mid} \end{cases}$$` -- A quick demo: ```r sort(X) ``` ``` ## [1] 151.6 151.8 153.7 156.9 161.8 163.3 164.9 165.8 167.4 176.0 ``` -- ```r median(X) ``` ``` ## [1] 162.6 ``` -- ```r mean(X) ``` ``` ## [1] 161.3 ``` --- layout: true class: bg-main3 split-30 # Standard Deviation - In a simple term, .amber[deviation] `\(d_i\)` tells you how far a data element `\(i\)` is from your mean value - So, we can calculate deviation as `\(d_i = X_i - \mu\)` - **However,** the deviation can be either *negative* or *positive* -- .row[.content[ ]] .row[.content[ {{content}} ]] --- Take our data as an example: ```r set.seed(1) *X <- rnorm(10, mean=160, sd=10) print(X) ``` ``` ## [1] 153.7 161.8 151.6 176.0 163.3 151.8 164.9 167.4 165.8 156.9 ``` -- ```r d <- X - mean(X) print(d, digits=2) ``` ``` ## [1] -7.59 0.51 -9.68 14.63 1.97 -9.53 3.55 6.06 4.44 -4.38 ``` -- Hard to find its general property! -- `\(\to\)` Potential solution? --- count: false We can take the **absolute** value and compute the mean: `$$\bar{d} = \frac{1}{N} \displaystyle \sum_{i=1}^{N} |X_i - \mu|$$` -- A quick demo: ```r d <- abs(X - mean(X)) print(d, digits=2) ``` ``` ## [1] 7.59 0.51 9.68 14.63 1.97 9.53 3.55 6.06 4.44 4.38 ``` ```r d.bar <- mean(d) print(d.bar, digits=2) ``` ``` ## [1] 6.2 ``` -- Now, it's easier to report your findings as `\(\bar{x} \pm \bar{d}\)` -- , or numerically as .amber[161.32] `\(\pm\)` .red[6.23] -- `\(\to\)` Yet, such a practice is uncommon to see. --- count: false Another alternative is to find the **root-mean square**, which define a .amber[standard deviation]: `$$\sigma = \sqrt{\frac{1}{N} \displaystyle \sum_{i=1}^N (X_i - \mu)^2}$$` -- A quick demo: ```r std.dev <- sqrt(sum({X - mean(X)}^2) / length(X)) print(std.dev) ``` ``` ## [1] 7.4 ``` --- count: false In statistics, we need to adjust the estimation by applying .amber[Bessel's correction]. -- Simply said, we find the mean by dividing into `\(n-1\)` instead of `\(N\)`. `$$s = \sqrt{\frac{1}{n-1} \displaystyle \sum_{i=1}^n (x_i - \bar{x})^2}$$` -- A quick demo: ```r *std.dev <- sqrt(sum({X - mean(X)}^2) / {length(X) - 1}) print(std.dev) ``` ``` ## [1] 7.8 ``` ```r sd(X) # Built-in function to calculate standard deviation ``` ``` ## [1] 7.8 ``` ??? Bessel's method applied to correct the bias in estimating population variance. --- layout: false class: bg-main3 # Variance - Another measure to estimate deviation - Akin to standard deviation, but without a root square -- - Computed as follow: `$$s^2 = \frac{1}{n-1} \displaystyle \sum_{i=1}^n (x_i - \bar{x})^2$$` -- **Importance:** - In making further inference - More advanced statistical model -- (outside the scope of this lecture, sorry!) --- name: quantile class: bg-main3 # Quantile - A .amber[cut-off] from a given probability distribution - Divide the data into a .amber[continuous] range -- **Our data:** ```r sort(X) ``` ``` ## [1] 152 152 154 157 162 163 165 166 167 176 ``` --- template: quantile class: split-two .column[.vmiddle.right.content[ 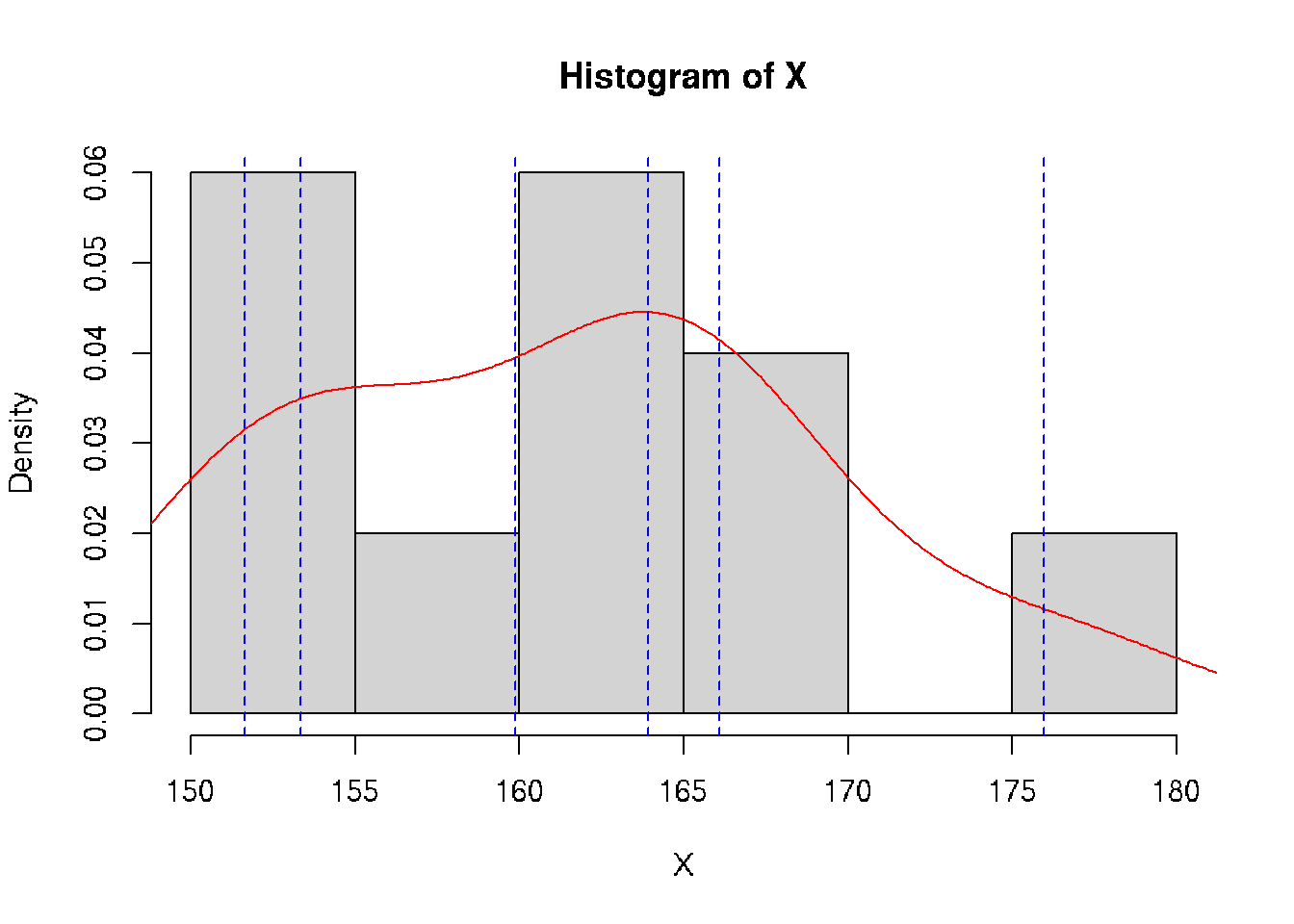<!-- --> ]] .column[.vmiddle.content[ ```r quantile(X, probs=seq(0, 1, 1/5)) ``` ``` ## 0% 20% 40% 60% 80% 100% ## 152 153 160 164 166 176 ``` ]] --- template: quantile class: split-two Quartile: A special case of quantile .column[.vmiddle.right.content[ 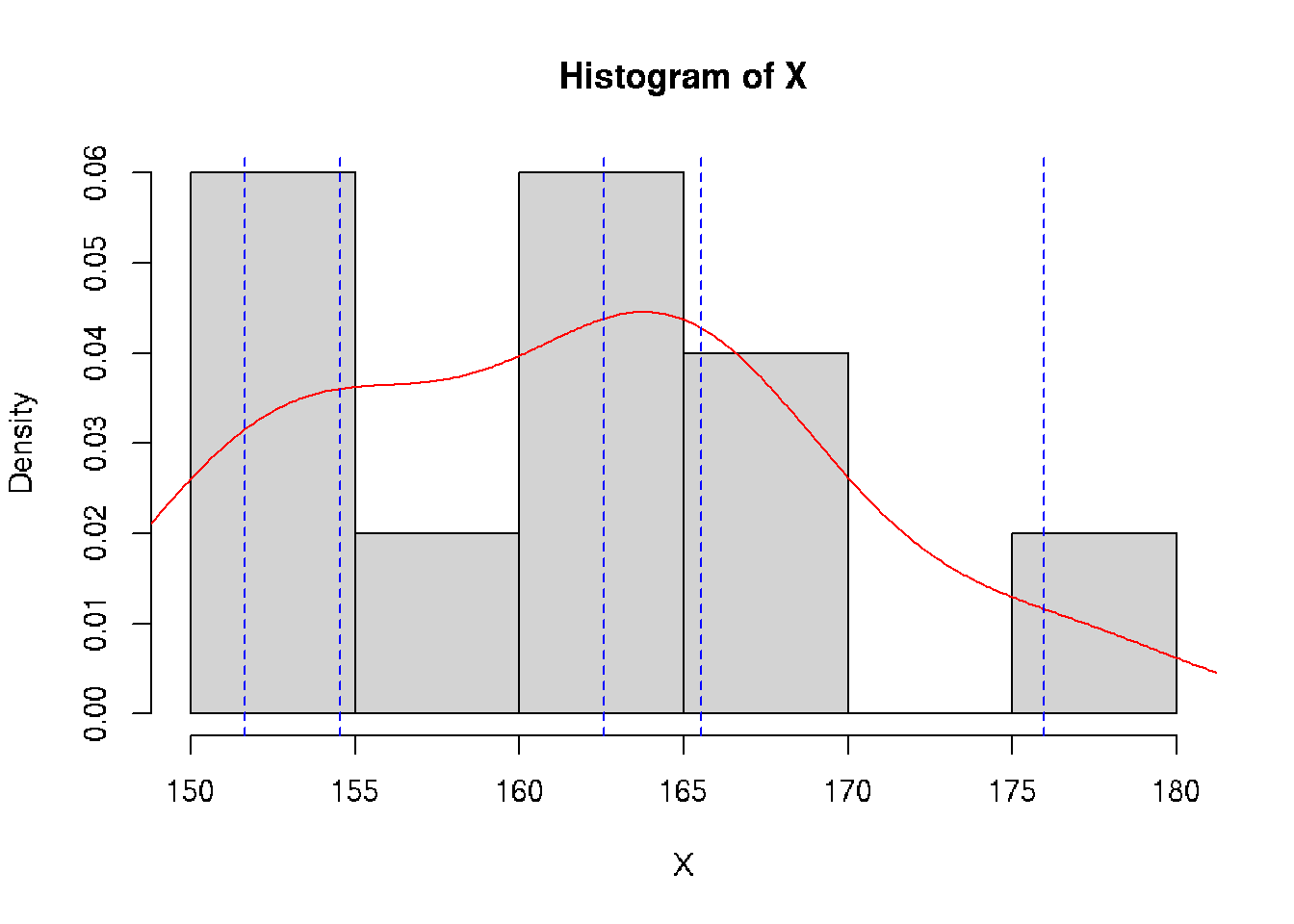<!-- --> ]] .column[.vmiddle.content[ ```r quantile(X, probs=seq(0, 1, 1/4)) ``` ``` ## 0% 25% 50% 75% 100% ## 152 155 163 166 176 ``` ]] --- layout: false class: bg-main3 split-two .column[.vmiddle.right.content[ .font5.amber[Conclusion] ]] .column[.font2.content.vmiddle[ - .amber[Central tendency:] - Mean - Median - .amber[Spread:] - Standard deviation - Variance - Quantile ]] --- class: bg-main3 split-two .column[.vmiddle.center.content[ <img src="https://blog.optimizely.com/wp-content/uploads/2015/02/stats-cat.jpg" width="100%"> ]] .column[.vmiddle.left.content[ .font5.amber[Query?] ]] --- name: sally-clarke layout: true class: bg-main3 split-two .column.bg-white[.vmiddle.center.content[ <img src="https://i.dailymail.co.uk/i/pix/2008/09/21/article-1059095-02BF33FE00000578-967_468x482.jpg" width="100%"> ]] .column[.vmiddle.content[ # Sally Clarke {{content}} ]] --- template: sally-clarke - December **1996**, home alone with her newly born baby - Suddenly the baby stopped responding, Sally called the ambulance - Failed to resuscitate `\(\to\)` Pronounced dead, diagnosed as SIDS - January **1998**, the same thing happened to her second child - Post-mortem: Retro-orbital and spinal bleeding --- template: sally-clarke count: false - UK criminology: two infant murders in the same household is 1 in 2mil - An extremely rare chance, indeed --- template: sally-clarke count: false - UK epidemiology: SIDS is about **1 in 8,500** healthy newborns - Probability of having two consecutive cases in a row? - Assuming independence, two consecutive cases may happen in 1:72mil - An even rarer case! --- name: malcolm-collins layout: true class: bg-main3 split-two .column.bg-white[.vmiddle.center.content[ <img src="https://image.freepik.com/free-photo/black-guy-blonde-woman-texting_102671-4270.jpg" width="100%"> ]] .column[.vmiddle.content[ # Malcolm Collins {{content}} ]] --- template: malcolm-collins - In 1964, a young lady snatched Mrs. Juanita Brooks' purse - According to eye witnesses: - The culprit was a woman in mid 20s - Had light blond hair with a pony tail - Went to a yellow motor car, driven by a black American - The guy had beard and mustache (**Disclaimer:** Photo is just an illustration) --- template: malcolm-collins count: false The chance of: - Black man with beard: **1 in 10** - Man with mustache: **1 in 4** - White woman with pony tail: **1 in 10** - White woman with blond hair: **1 in 3** - Yellow motor car: **1 in 10** - Interracial couple in car: **1 in 1,000** Overall probability of consecutive independent occurrence: **1 in 12mil** `\(\to\)` Rare! --- layout: false class: bg-main3 font2 # .amber[Similarities] of both cases? - Both relied on .amber[probability] theory. - Basically: .amber[statistics] -- ... More or less. -- - Used in .amber[court room] to determine innocence. -- - ... And both were .red[**grave mistakes**]. -- - Let's quickly reinvestigate both cases :) --- template: sally-clarke - The occurrence of SIDS is not independent - Meaning that, there is a higher likelihood of having a second child affected by SIDS - ... If your first child is - Since they are not independent, multiplying the proportion would not do justice to estimate the chance --- template: malcolm-collins - Just like in Sally Clarke's case - The chance of finding a black guy with beard and mustache... (and so on, as previously described)... Is not an independent event --- class: bg-main3 # Then, is statistics .red[wrong]? .font2[ - Not always the case ] -- .font2[ - Sometimes, we unconsciously obscure the fact with numbers ] ??? Example: 8 among 10 dentists recommend Colg*te -- .font2[ - Now we will learn some examples where statistics help telling the truth ] --- class: bg-main3 # How .amber[statistics] describe .red[mental health] .font2[ - .amber[28%] of HIV-positive participants were having .red[depressive] symptoms `\(^1\)` - .amber[49%] of the French neurosurgical community reported .red[burnout] `\(^2\)` - .amber[9.4%] of medical students in a study had .red[suicidal ideation] within the past 12 months `\(^3\)` ] <br> <br> <br> <br> <br> <br> .font1[ 1. S. K. Y. Choi, E. Boyle, J. Cairney, et al. .amber[“Prevalence, Recurrence, and Incidence owe Current Depressive Symptoms among People Living with HIV in Ontario, Canada: Results from the Ontario HIV Treatment Network Cohort Study”]. In: _PLOS ONE_ 11.11 (Nov. 2016). Ed. by V. D. Lima, p. e0165816. DOI: 10.1371/journal.pone.0165816. 1. C. Baumgarten, E. Michinov, G. Rouxel, et al. .amber[“Personal and psychosocial factors of burnout: A survey within the French neurosurgical community”]. In: _PLOS ONE_ 15.5 (May. 2020). Ed. by S. A. Useche, p. e0233137. DOI: 10.1371/journal.pone.0233137. 1. L. N. Dyrbye, C. P. West, D. Satele, et al. .amber[“Burnout Among U.S. Medical Students, Residents, and Early Career Physicians Relative to the General U.S. Population”]. In: _Academic Medicine_ 89.3 (Mar. 2014), pp. 443-451. DOI: 10.1097/acm.0000000000000134. ] --- class: bg-main3 middle center .font3[Slide and short note: http://bit.ly/biostatistik-ukrida]